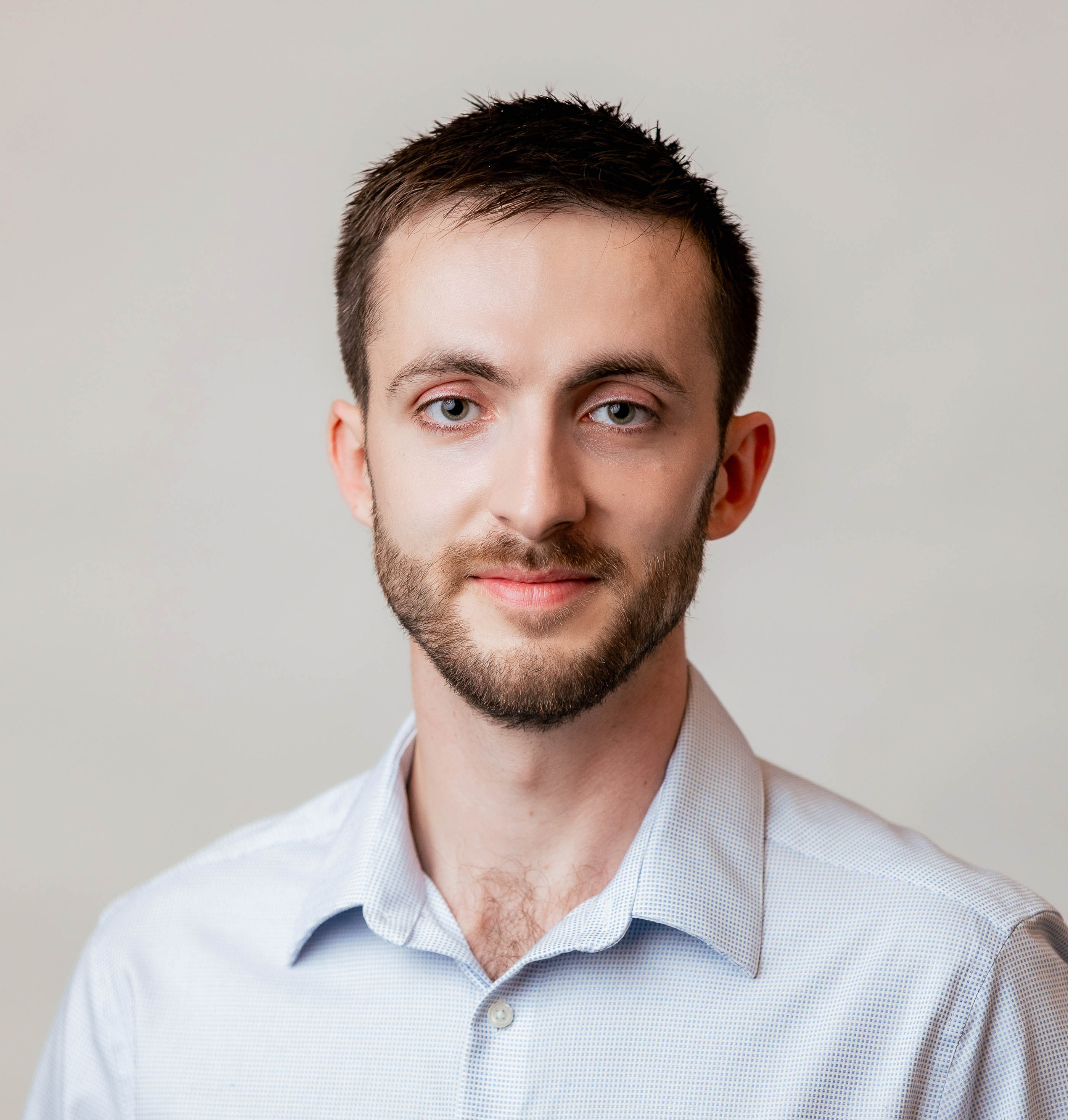
Jake A. Soloff
I am a postdoctoral researcher at the University of Chicago, broadly interested in the foundations of data science and machine learning. In my research, I develop theoretical frameworks that enable strong statistical guarantees without restrictive assumptions, allowing practitioners to apply rigorous tools with minimal tuning and maximal flexibility.
Current Position
Postdoctoral Researcher in Statistics
University of Chicago
Advisors: Rina Foygel Barber & Rebecca Willett
Education
Ph.D. in Statistics (2022)
University of California, Berkeley
Advisors: Aditya Guntuboyina & Michael I. Jordan
Select Publications
Building a stable classifier with the inflated argmax
J. A. Soloff, R. F. Barber, and R. Willett
Advances in Neural Information Processing Systems 37 (NeurIPS 2024)
Bagging provides assumption-free stability
J. A. Soloff, R. F. Barber, and R. Willett
Journal of Machine Learning Research, 25(131), 1-35
The edge of discovery: Controlling the local false discovery rate at the margin
J. A. Soloff, D. Xiang, and W. Fithian
Annals of Statistics, 52(2), 580-601
Multivariate, heteroscedastic empirical Bayes via nonparametric maximum likelihood
J. A. Soloff, A. Guntuboyina, and B. Sen
Journal of the Royal Statistical Society: Series B, 87(1), 1-32
Preprints
Can a calibration metric be both testable and actionable?
R. Rossellini, J. A. Soloff, R. F. Barber, Z. Ren, and R. Willett
Cross-validation with antithetic Gaussian randomization
S. Liu, S. Panigrahi, and J. A. Soloff
Stabilizing black-box model selection with the inflated argmax
M. Adrian, J. A. Soloff, and R. Willett
Testing conditional independence under isotonicity
R. Hore, J. A. Soloff, R. F. Barber, and R. J. Samworth
Stability via resampling: Statistical problems beyond the real line
J. A. Soloff, R. F. Barber, and R. Willett
Covariance estimation with nonnegative partial correlations
J. A. Soloff, A. Guntuboyina, and M. I. Jordan
Incentive-theoretic Bayesian inference for collaborative science
S. Bates, M. I. Jordan, M. Sklar, and J. A. Soloff